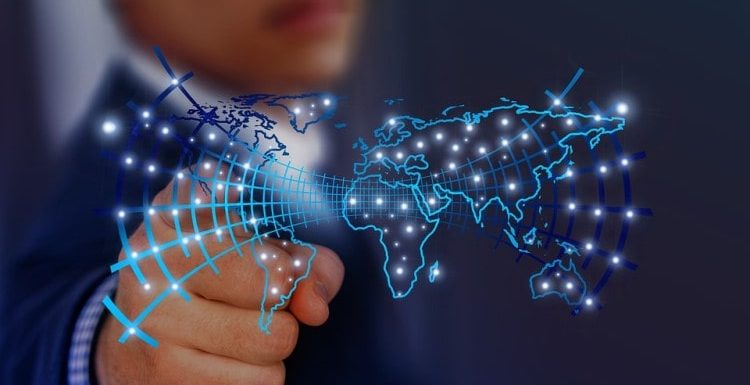
The ever-changing landscape of technology is flooding the job market with new opportunities for Data professionals. We see it every day – data science, data engineering, and big data are everywhere! Which of these roles should you pursue if you’re looking for a career with better pay and more opportunity? Data scientists or data engineers? Which has high scope?
The answer is not clear-cut. While both roles demand significant skill sets, some might have a natural advantage over the other. This article explains the differences between a data scientist and a data engineer and then helps you decide which one might be the best fit for you.
Data Scientist vs. Data Engineering: What’s The Difference?
There is no question that data science and data engineering are two different fields with two different skill sets. However, it’s important to remember that both disciplines are analytical in nature. Data scientists focus more on statistical ways to deal with the data, while a career as a data engineer will require you to have a strong understanding of mathematical concepts and computer science knowledge.
A data engineer will typically have a job outside of the organization’s core, perhaps reporting and analyzing data from an outside source. A data scientist will work directly with the company’s employees and customers, taking specific and actionable steps based on the information at hand.
So, let’s take a look at what a data scientist and a data engineer do.
Who is a Data scientist?
A data scientist focuses on using data to solve problems supplied by a data engineer. He performs statistical analysis, creates machine learning models, and applies artificial intelligence techniques. They also have excellent communication skills to present their findings to business leaders or customers. Data scientists use complex algorithms to figure out what types (or categories) of data exist within their dataset and then use those categories to make predictions about future events.
Skills needed to become a data scientist:
-
Programming languages:
A data scientist must be proficient in programming languages, especially R and Python. But, Python has recently emerged as a popular choice among data scientists. These programming languages make it easier and faster for data scientists to draw conclusions from massive datasets. Apart from this, these programming languages help data scientists organize unstructured datasets.
-
Strong statistical and mathematical skills
Data scientists must be highly proficient in mathematics and statistics. Even when developing machine learning algorithms, the core concepts of statistics must be strong. Hence, they must be well-versed in probability distributions, hypothesis testing, etc.
-
Business Acumen:
A data scientist is responsible for addressing the organization’s business issues and proposing strategies to improve them. Thus, strong business acumen is the most effective means of channeling technical skills profitably. Without it, an aspiring data scientist may not be able to recognize the problems and potential obstacles that must be addressed for a business to grow.
-
Knowledge of Analytical Tools:
Analytical techniques are useful for collecting relevant information from a well-structured dataset. Analytics tools such as SAS, Hadoop, Spark, Hive, Pig, and R are the most commonly used by data scientists. Through various certifications like data scientist courses, you can learn and demonstrate your expertise in these analytical tools.
Who is a Data Engineer?
Data engineers build tools that help other people collect new types of data so they can then analyze it using their algorithms. They also build tools that allow users to visualize their findings in different ways to understand them better.
For example, an engineer might write code that enables users to see all their social media posts on one screen at once instead of scrolling through each one individually.
In other words, a data engineer’s primary concern is building the infrastructure to process and store large data. They may also develop algorithms for automated processes that run on top of databases. Still, these are secondary tasks for them compared with building and maintaining hardware/software systems that efficiently handle huge volumes of data.
Skills needed to become a data engineer:
-
Database architecture and data warehousing
As the name suggests, a data warehouse stores vast data for analysis. Hence, a data engineer is expected to have a solid understanding of data warehousing fundamentals and the tools that go along with them.
-
ETL Tools
ETL (Extract, Transfer, Load) assists in the extraction and transformation of data so that it can be analysed. These ETL tools help data engineers gather data from various sources, convert it to a usable format, and store it in a database for usage by analytics experts within the organization. Thus, a data engineer must have a firm grasp of these tools.
-
Knowledge of data structures
A data engineer is also required to be well-versed in data structures. It assists them in understanding the organization’s business goals and delivering solutions based on those goals.
-
Programming Languages
Data engineers must also be familiar with programming languages such as Python and Java, with Python being the most in-demand. Strong coding abilities enable data engineers to work on many computer languages that a company utilizes to develop its pipelines.
Salary outlook of data scientists and data engineers:
Generally, data engineers’ and data scientists’ salaries are determined by the type of role, relevant experience, and job location. According to Glassdoor, the average annual pay scale for a data engineer is around $142,000, while that of a data scientist is approximately $139,000.
Can data scientists transition to data engineers?
Yes. With some further training, data engineers can become data scientists and vice versa. Because of the overlap in abilities – from knowledge of programming language to work with data pipelines – individuals of both professions have the underlying knowledge and terminology to make a relatively smooth career shift.
Upgrade your skills with a Data science course in Pune to make a career transition as a data scientist, data engineer, or even a data analyst.
So, which one would be a better choice for you?
Careers as data scientists and data engineers are both fulfilling in their own ways. Because of the inherent complexities of each role, there’s not a clear answer to which a better career choice is. However, if you enjoy building algorithms, architecture, and infrastructure, data engineering sounds like a better option. But, If you are interested in business or developing hypotheses, then a career as a data scientist might be more fitting for you.
It all comes down to your passions and strength.
Summary:
The differences between these two popular roles are exemplified in their respective specialties. To summarize, Data scientists get to delve into the world of statistics, machine learning, and advanced data analysis, while data engineers are tasked with the more technical aspects of designing and building platforms with ever-evolving data architectures.
Both have their own sets of strengths and weaknesses, and you should be aware of those before deciding on your next career move. Whatever you choose, keep in mind that “Passion for the work will get you farther than technical skills.“
Author bio:
I am Keerthika and I am a passionate blogger who loves to write educational and technical content about data science courses, Machine Leaning and Artificial Intelligence. I do my best writing in each and every aspect of data science. I always trust the learning process in a smart way so that people will understand the concept in a better way. The only thing I admire about people is how they value the learning time that will make me put more effort on them. I always prefer the writings which will help them to succeed and build up their business.